Advantages and Disadvantages of Generative AI in Healthcare
Updated: May 10, 2024
82
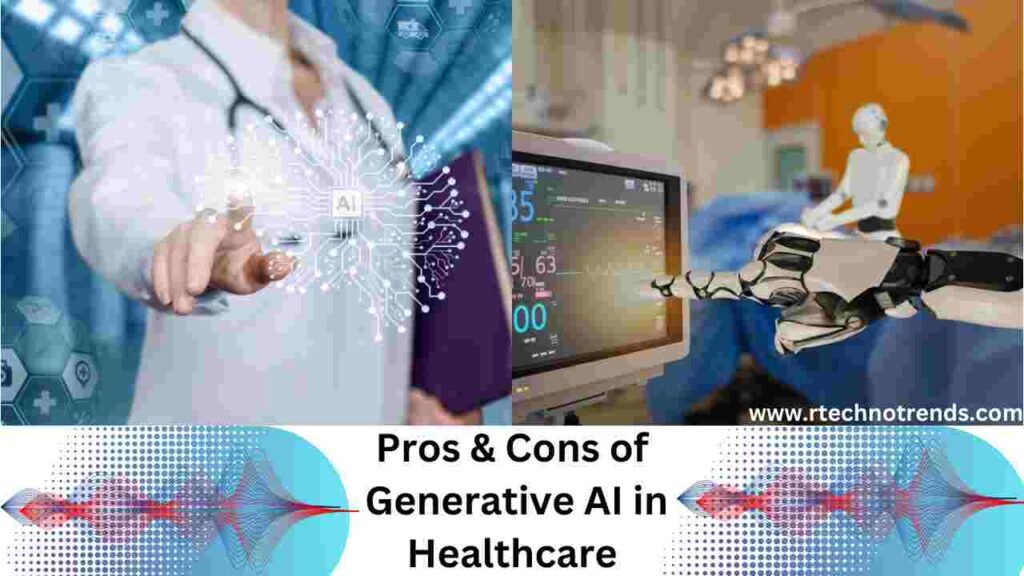
Generative AI has the potential to transform the healthcare industry by providing patients, doctors, health insurers, and other healthcare providers with powerful tools that can analyze medical data and provide more effectiveness in diagnosing some chronic illnesses and personalized treatment plans. However, a machine is after all a machine. This renders there is ample room for improvement in utilizing generative AI within healthcare and we should consider the risks that the individuals and entities face when implementing this.
Advantages & Disadvantages of Generative AI in Healthcare
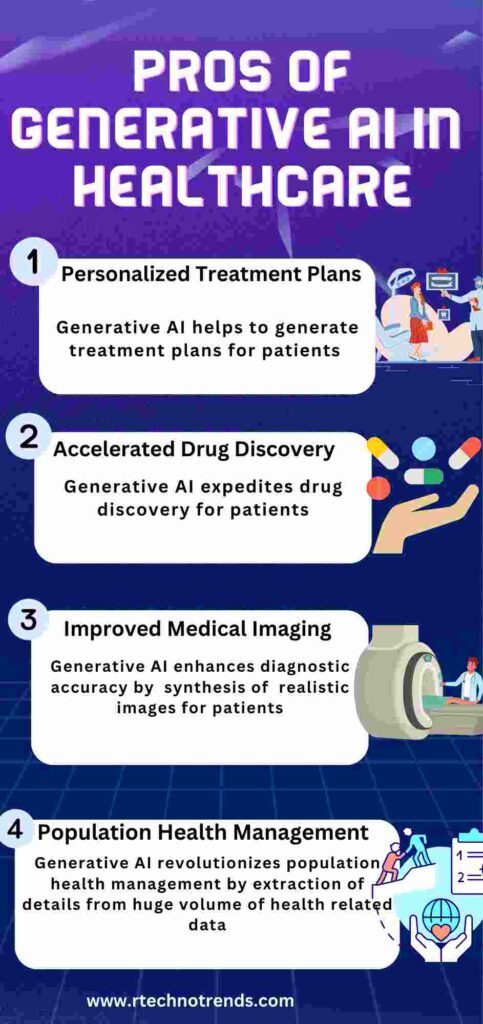
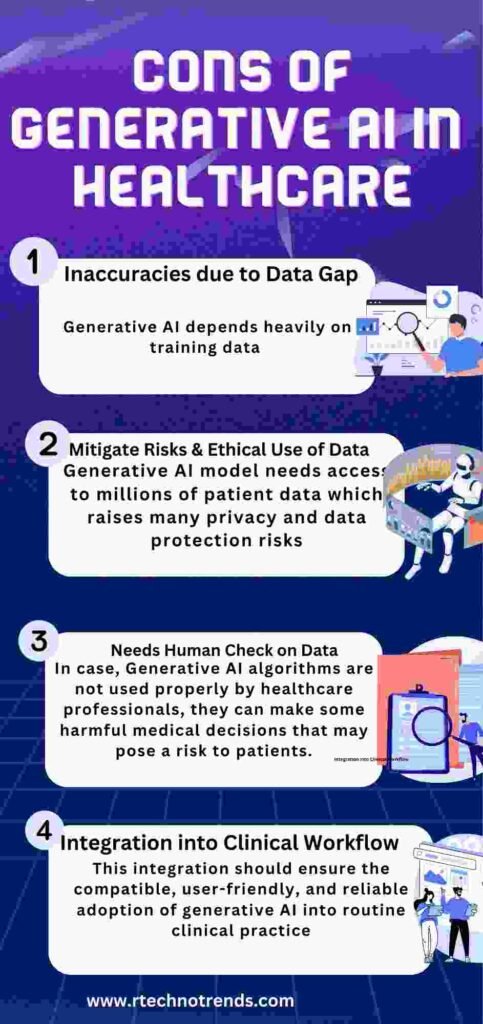
Advantages of Generative AI in Healthcare
- Provide Personalized Treatment Plans
Generative AI helps to generate treatment plans for patients by analyzing the following patient’s unstructured data that includes:
- Medical records
- Genetic information
- Lifestyle factors
- Audio recordings of consultations
- Clinical treatment history.
Generative AI in healthcare makes use of machine learning algorithms to identify patterns and correlations within the patient’s medical data and develop personalized treatment strategies.
These multiple data points in generative AI models assist healthcare professionals in making data-driven decisions, ensuring that patients receive the most appropriate and effective treatment options available.
2. Accelerated Drug Discovery and Development
Traditional drug discovery involves synthesizing and testing numerous compounds which is a time-consuming and expensive process. Whereas AI (machine learning models) expedites drug discovery by
- Simplifies the process of finding and confirming potential molecular targets by analyzing various datasets.
- Suggesting novel compounds based on existing data.
- It swiftly identifies and flags any potential safety concerns ensuring patient safety and well-being.
- Evaluating drug’s effectiveness through the process of computer simulations before progressing to clinical trials on animals and humans.
3. Improved Medical Imaging
One of the biggest contributions of generative AI in healthcare is into medical imaging. It makes use of two generative models such as
Generative Adversarial Networks (GANs) — A generator network that creates synthetic images and a discriminator network that is used to differentiate between real and synthetic images.
Variational Autoencoders (VAEs) – It has received considerable attention. It learns data from a low-dimensional representation of an input image and then generates new images by randomly sampling from this latent space.
One of the biggest contributions of generative AI in healthcare is into medical imaging. It makes use of two generative models such as
Deep learning algorithms in generative AI:
- Can automatically identify any abnormalities in CT or MRI images and analyse doctors for any potential risks to the patients.
- Are trained on synthetic data that enables the generation of synthetic images that closely resemble real patient scans.
- Enhances diagnostic accuracy by synthesis of realistic images and other reconstruction techniques that enable healthcare professionals to analyse images with greater precision and confidence.
4. Population Health Management
A) Generative AI models revolutionize population health management by extracting details from a huge volume of health-related data including:
- Electronic health records
- Insurance claims
- Social determinants of health
- Remote device data
- Public health records
B) After extraction AI algorithms
- Analyse this diverse data.
- Identify correlations!
- Reveal patterns that are otherwise challenging to detect.
As a result, AI models can assist in the
- Prediction of future health outcomes
- Stratifying risk levels
- Identifying communities that can benefit from some preventive health measures
Disadvantages of Generative AI in Healthcare
1) Inaccuracies due to Data (Gap & Bias)
Generative AI models depend heavily on the training data available from millions of catalogued cases. If the training data is biased or missing for example the prescriptions of a certain population may be absent, then the generated images may inherit these biases which may lead to inaccurate diagnoses or required treatment. AI constantly evolves and improves to account for such data gaps.
2) Mitigate Risks & Ethical Use of Patient Data
Generative AI model needs access to millions of patient data that raises many privacy and data protection risks. It’s tough to maintain patient confidentiality and obtain consent for data usage for security reasons. The solution to it is:
- Implementing robust data governance frameworks
- Complying with legal and regulatory requirements
- Improved cyber security to ensure technology is risk free
3) Needs Human Check on Data
Although AI has many benefits in the medical world, there is always a need for human checks. Take for example the surgery robots operate logically without any empathy with the patient. Generally, doctors may observe some vital behavioral changes in a patient that can help to diagnose any underlying medical complication.
In case, generative AI algorithms are not used properly by healthcare professionals, they can make some harmful medical decisions that may pose a risk to patients. Or else there is a chance of healthcare providers being over-reliant on these algorithms that they lose their power to make independent medical judgments.
4) Integration into Clinical Workflow& Electronic Health Records
The ml and ai algorithms hold utmost significance in addressing important clinical workflow when it is integrated into medical imaging systems.
What is required for this?
Healthcare providers need continuous integration of generative AI tools into
- Their imaging systems
- Electronic health records
- Picture archiving and communication systems (PACS).
This integration should ensure the compatible, user-friendly, and reliable adoption of generative AI into routine clinical practice.
Conclusion:
AI can undoubtedly improve healthcare systems. It can track real-time data that can better and more rapidly do the disease diagnosis. Generative AI technology is more applicable and better informed. They can surely reduce many administrative errors and save many vital resources. Despite some of the drawbacks and limitations of AI, this innovative technology is still extensively being used due to its extraordinary benefits to the medical sector.
What are the risks or challenges of generative AI in healthcare?
Generative AI in healthcare depends on vast amounts of data which is the sensitive patient information used to train the models and generate insights. The major challenges of AI in healthcare are data privacy and security risks.
Please Write Your Comments